Understanding Recommendations
Generating Recommendations
Slingshot analyzes warehouse trends to find areas of optimization for your warehouses based on idleness, query load, queueing, spillage, and utilization. Slingshot identifies 1 of 11 possible issues.
Potential Warehouse Issue | Definition |
---|---|
High auto-suspend | When warehouses run for long periods of inactivity before suspending activity |
High idleness | When a warehouse consumes credits without executing any queries for long periods of time |
High query load | Several queries run at once, reduces performance and strains compute resources |
High average (avg.) queue | A large number of queries waiting to be processed, impacts overall warehouse performance |
High spill | Queries that require additional compute resources and rely on spilling data to remote storage for execution |
Long query | Queries take a substantial amount of time to complete due complexity, size, or variety of factors |
Low query load | An underutilized warehouse that runs minimal queries |
Low average (avg.) queue | An underutilized warehouse with only a few tasks waiting in queue |
Low spill | A warehouse with capacity to run more queries, it’s executing without spilling to remote storage |
No auto-suspend | During periods of inactivity the warehouse continues to run and consume credits |
No warehouse load | An inactive warehouse, underutilized |
Short query | Queries execute quickly, underutilized |
Hourly analysis on each warehouse looks for a single issue or a combination of issues to initiate a recommendation. There are 17 possible issue combinations. Combinations consist of a single issue or two or more issues together.
For example, a finding of High query load, Long query, High spill, High avg queue results in a recommendation to increase max cluster count.
Red tags on the Recommendation page identify the trigger and action. Dissect a Recommendation in Anatomy of a Recommendation.
Recommendation | |
---|---|
Suggested action | Trigger (includes single issues and combinations) |
Decrease warehouse size | Null query Load |
Low query load, Short query, Low spill | |
Low query load, Short query | |
Low query load, Low spill | |
Low query load, Low avg queue | |
Increase max cluster count | High query load, High avg queue |
High query load, Long query, High spill, High avg queue | |
High query load, Long query, High avg queue | |
Increase warehouse size | Long query, High spill |
Long query | |
High spill | |
Decrease warehouse size and Increase max cluster count | High query load, Short query, Low spill, High avg queue |
High query load, Short query, High avg queue | |
High query load, Low spill, High avg queue | |
Decrease auto-suspend | High idleness |
High auto-suspend | |
No auto-suspend |
Anatomy of a Recommendation
Review and adjust recommendations or apply them as is. The Recommendation consists of four parts.
- Why you’re seeing this recommendation: A summary of the Slingshot-identified Issues, current cost and performance info (Average query execution time and Monthly cost), and the Projected results (cost and performance) should you apply the recommendation as suggested without making changes.
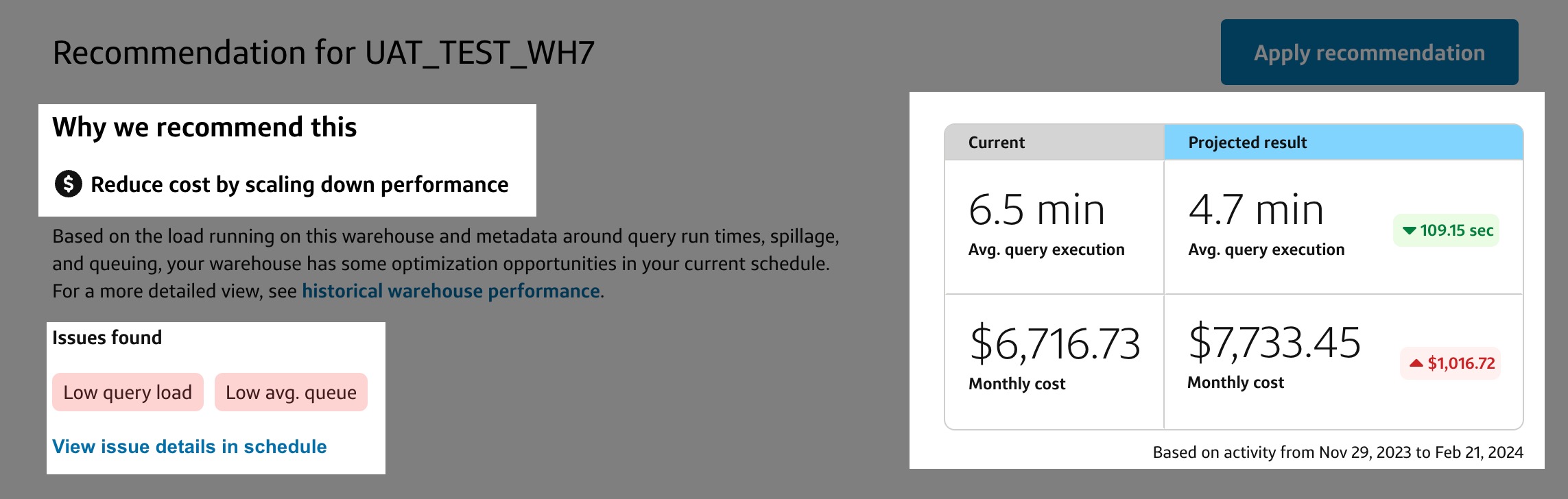
- What to optimize: The Recommendation settings and schedule
compare the current settings and schedule with the recommended
settings and schedule. Make adjustments to the suggested parameters or
settings. Learn more about editing recommendations, see Manage
Recommendations.
- Compare schedules: Toggle between the current and recommended schedules to see which time blocks need your attention based on Slingshot’s hourly analysis of your warehouse’s performance.
- The current schedule flags areas of opportunity in red with a flag
icon. Selecting any red time block displays an issue list and
explanation.
- How optimization happens: Review the Projected impact on
queries and Historical warehouse performance tabs to gain
insights about the impacts of the issues and how taking Slingshot’s
suggestions improves performance or costs. These tabs provide you with
valuable analysis tools and answers the key question, what happens
when I apply this recommendation.